How many completed sprints are needed for forecasting?
- Julia Wester
- Feb 18, 2022
- 2 min read
Updated: Jul 20, 2022
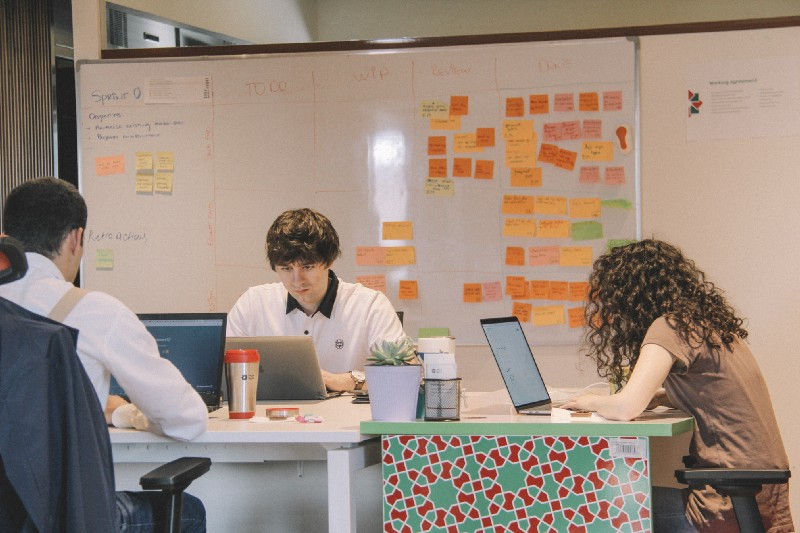
Probabilistic forecasting via Monte Carlo Simulations are a key feature of two of our products, ActionableAgile Analytics and Portfolio Forecaster. These simulations can provide amazingly accurate forecasts with a couple of clicks of a button, but there are lots of considerations when it comes to the data you use to generate the forecasts.
A customer recently asked us about one of these considerations and we thought it was worth sharing.
How many sprints are required to get decent data for a Monte Carlo sim?
Our tool warns you that your forecasts might be iffy if you have less than 10 data points (finished work items), regardless of the number of sprints you have.
Daniel Vacanti, creator of ActionableAgile and well-known trainer and speaker on metrics, goes into a little more detail. He says “you can forecast once you have approximately 10 data points regardless of the number of sprints. However, the quality of the forecast you will get is rather dependent on how good of flow you have during the sprint. If all items finish on the last day, then you may need several sprints before you can make a good forecast. However, in that case your biggest problem isn’t the data, it is how you are running your process.”
The underlying consideration
The rule of thumb with Monte Carlo simulations is that the conditions that were present when you generated your past data are roughly similar to the conditions that will exist during the period you are attempting to forecast. The word “conditions” here can refer to many different factors: team capacity, the mix of work, amount of work-in-progress, etc. These will all impact your throughput and throughput is the data used to forecast via our Monte Carlo simulations.
So, if you have 10 items and that 10 items roughly represents the mix of work you’ll be doing in the future, those 10 items may be sufficient. However, if you have more cyclical work items or other considerations, you might need a bigger data set that accounts for that variety.
Improve forecasts by improving predictability
The less predictable you are — meaning the bigger the variation in how long it takes you to deliver work and how many work items you deliver over time — the more data you might need to get a better forecast.
If you aren’t happy with your forecasts, usually the answer lies in improving predictability by controlling the age of in progress items. The more you prevent unnecessary ageing, the better your predictability.
Want to try out probabilistic forecasting? Try ActionableAgile Analytics for free whenever you’re ready.
Comments